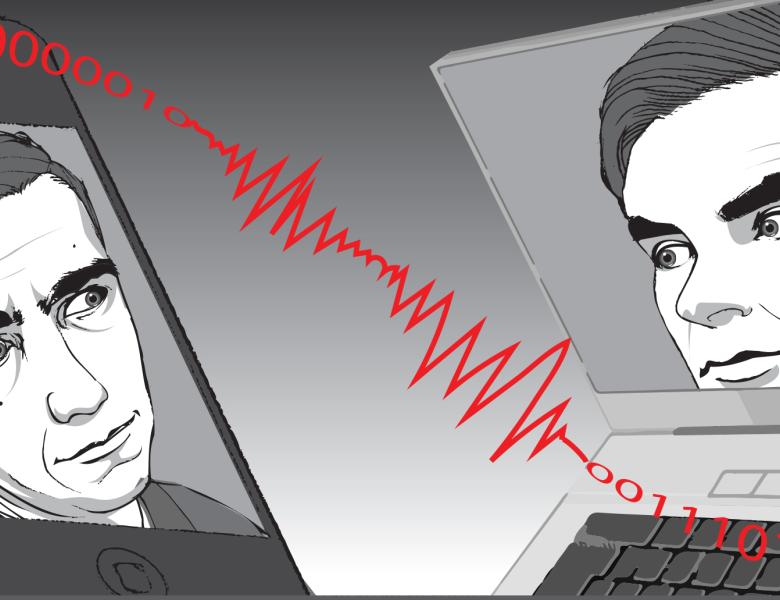
Abstract
We propose a general approach to estimating functionals of discrete distributions, and illustrate its efficacy by analyzing the cases of estimating the Shannon entropy H(P) and the alpha-moment of the distribution, based on n i.i.d. samples from the unknown discrete distribution P with support size S. The resulting estimators achieve the minimax L2 rates in all regimes, including those where the support size S grows with n. They have linear complexity and do not assume knowledge of S. The performance of these estimators with n samples turns out to be essentially that of the respective Maximum Likelihood Estimators (MLE) with n log n samples. Approximation theory plays key roles in analyzing the MLE (the plug-in rule for functional estimation), and in both the construction and analysis of the minimax rate-optimal estimators. As corollaries, we show how our results are consistent with other recent results, such as the optimal sample complexity n = \Theta(S/log S) for entropy estimation, first discovered by Valiant and Valiant in 2011.
Furthermore, we show that our entropy estimator is an adaptive estimator in the sense that it achieves the minimax rates simultaneously over a nested sequence of subsets of distributions P, without knowing the support size S or which subset P lies in. Each subset is characterized by an upper bound on the distribution entropy. We also characterize the maximum risk of the MLE over this nested sequence, and show, for every subset in the sequence, that the performance of the minimax rate-optimal estimator with n samples is still essentially that of the MLE with n log n samples.
We discuss the extension of our approach to estimating divergence functions, such as the total variation distance. We demonstrate that the construction is far more complicated than the univariate case, and showcase a general interpretation of our methodology in estimating functionals in arbitrary statistical models.
We highlight the practical advantages of our schemes for the estimation of entropy and mutual information. We compare our performance with various existing approaches, and demonstrate that our approach reduces running time and boosts the accuracy. Moreover, we show that the minimax rate-optimal mutual information estimator leads to significant performance boosts over the Chow–Liu algorithm in learning graphical models. Given the wide applicability of information measure estimation, we believe that the new insights and near optimal estimators have the potential for far reaching applications.
Based on joint work with Kartik Venkat (Stanford EE), Yanjun Han (Tsinghua University), and Tsachy Weissman (Stanford EE).