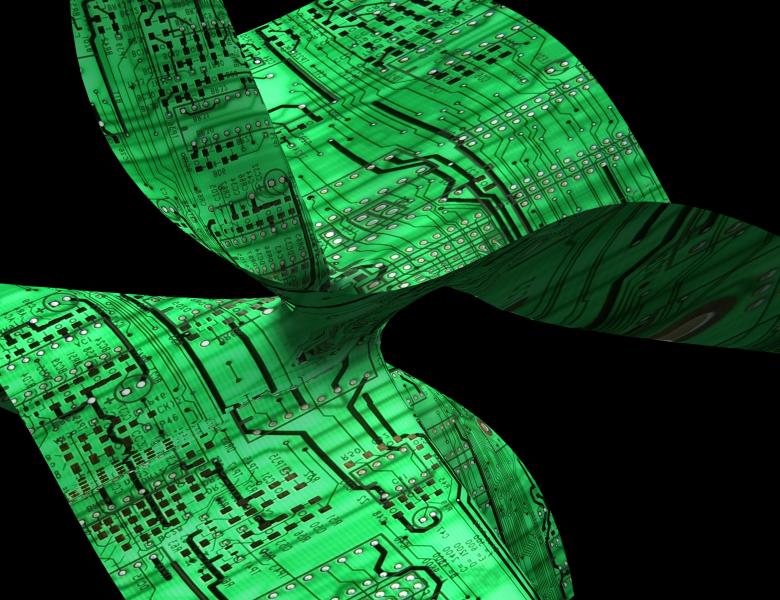
Abstract
The tensor decomposition problem can be seen as a special instance of the reconstruction problem of sum of exponential functions from truncated moment sequences. This sparse representation problem appears in many applications.
We recall an approach due to Prony for solving the problem in the univariate case, and describe an extension to the multivariate case. The method is illustrated on several examples coming from signal processing, magnetic resonance imaging, sparse interpolation, polynomial optimization, When few moments are known, we would like also to be able to find a sparse decomposition.
This problem corresponds to a flat extension property, which reduces either to a rank completion problem on structured matrices or to solving commutation constraints.
We analyze some of its geometric properties, describe some techniques to solve it and present some applications.