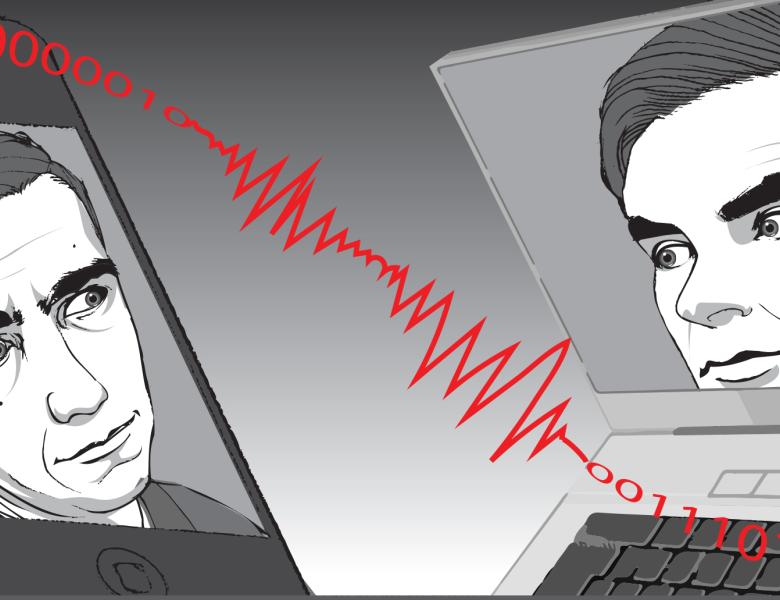
Abstract
PAC (proper) learning estimates a distribution in a class by some distribution in the same class to a desired accuracy. Using spectral projections we show that spherical Gaussian mixtures in $d$ dimensions can be PAC learned with $\tilde{O}(d)$ samples, and that the same applies for learning the distribution's parameters. Both results significantly improve previously known bounds.
Joint work with Jayadev Acharya, Ashkan Jafarpour, and Alon Orlitsky.